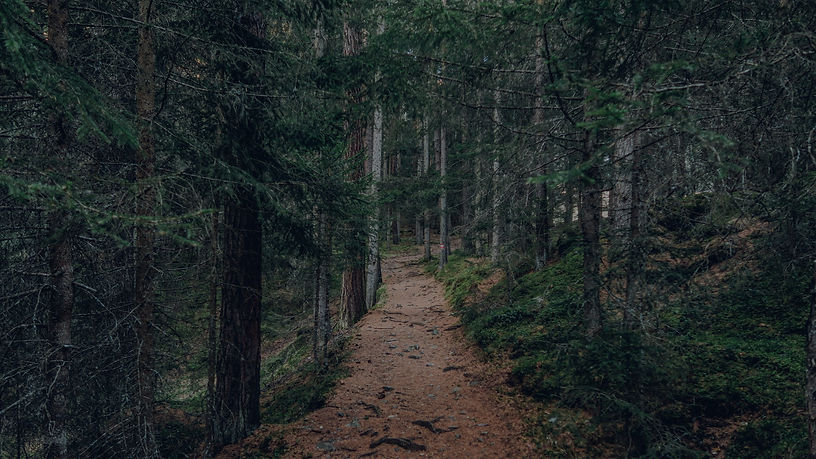
Rebate Analysis
Maryland Residential Clean Energy Rebate Program Analysis:
A socio-economic comparison of solar, geothermal and pellet stove awards
December 2020
by Melissa Bollman, PhD Candidate
Click here to download a PDF copy of the report
Table of Contents
Executive Summary
As the need to decarbonize the United States energy system grows, more state energy agencies are offering technology incentive programs to promote renewable energy generation, environmental quality, and regional development. Such programs are often evaluated on the principle of additionality—did the program result in more low-carbon energy generation than would have occurred in its absence?—but an another metric to consider is justice—did the program serve different communities and regions equitably?—because the need to decarbonize the country rapidly must be balanced with the need to reduce socioeconomic inequality.
​
This research focused on these metrics by analyzing the rebate distribution patterns of two Maryland Energy Administration (MEA) residential renewable energy rebate programs: The Residential Clean Energy Rebate Program (active 2005 - present) and the Clean Burning Wood and Pellet Stove RebateProgram (active 2012 - present). MEA program data collected over a period of 15 years (February 2005-June 2020) was used to compare how the distribution of low-carbon technology incentives across multiple energy resource types—solar, geothermal, and woody biomass—correlate with various social and environmental variable distributions.
​
Of special interest was the magnitude of divergence in the distribution patterns of wood and pellet stove and solar and geothermal rebates, given the large gap in cost for these systems. The MEA's programs use a tiered award structure, wherein solar photovoltaic and geothermal awards are more than two to three times higher than awards for wood and pellet stoves. Because MEA programs do not exclude high earning households nor award larger rebates to low-income households, we expected more rebates for higher cost technologies to be claimed by wealthier households. Moreover, we predicted that the differing physical characteristics of renewable energy systems would influence their regional adoption rate. For example, we expected wood stoves would be more popular in areas where firewood is more readily available.
​
Quantitative spatial analysis techniques were used to estimate the degree of correlation between the number of renewable energy incentives granted to a Maryland zip code and the presence of explanatory variables, including income, race, and forest cover, as well as control variables, such as the percentage of residents using renewable fuels. Rebate hot spots and cold spots were estimated using the Optimized Hot Spot Analysis function in ArcGIS 10.7 software. In addition, an ordinary least squares (OLS) regression model was created to assess the statistical relationship between rebate distribution patterns and explanatory and control variables.
​
Results of these analyses show that the program's rebate distributions patterns largely conform to expectations, but also exhibited some patterns that may be less intuitive, such as the degree of overlap between geothermal and woody biomass rebate hotspots despite the difference in the upfront costs of these technologies. Similarly, the OLS regression model did not uncover a statistically significant correlation between the number of solar and biomass rebates awarded in a zip code and average household income as expected. Future research could test whether these zip code-level trends hold for other spatial scales, such as the country or Census Tract. Examining how Maryland renewable energy award distributions compare to other state's technology rebate programs also would be useful for contextualizing the results.
Summary of Key Findings
This analysis used geographic information science (GIS) and statistical techniques to uncover trends in the distribution patterns of MEA's clean technology rebates over the past fifteen years. The study's key findings are as follows:
-
Solar technology rebates were more likely to be awarded in the central part of the state (Montgomery, Price George's, and Howard Counties) and less likely to be awarded in the Eastern Shore, Southern Maryland, or the Western Panhandle.
-
Wood and pellet rebates were more likely to be awarded northeast of Baltimore (Howard County) and eastern parts of Southern Maryland and less likely to be awarded in Montgomery and Howard Counties.
-
Geothermal rebates were more likely to be awarded in central, northeast, and southeast Maryland, and less likely to be awarded near Washington DC.
-
Solar and woody biomass grant hotspots had little overlap, while geothermal hotspots overlapped to a large degree with the wood and pellet hotspot in Harford County and the solar rebate hotspot in the central part of the state.
-
Solar rebates tend to be clustered in areas with high rates of solar heating (center of the state), while wood and pellet hotspots are located away from Western Maryland where wood heating is prevalent. (Note: The Census does collect locational data for homes that heat primarily with geothermal systems.)
-
Rebates of any technology type were less likely to be awarded to zip codes with a higher percentage of renters and older construction homes.
-
Rebates were more likely to awarded to zip codes with a greater percentage of Asian residents, higher electricity prices, and a higher number of warmer days.
-
Solar rebates were more likely to be found in urban areas than geothermal and woody biomass rebates. (Note: The Census' definition of ‘urban' is very broad and includes areas with 50,000 or more people.)
-
Wood and pellet rebates tend to cluster in more forested areas of the state, while solar rebates tend to cluster in less forested areas. Geothermal rebates also tend to be found in areas with more forest cover.
-
Geothermal rebates are more likely to be awarded to zip codes with higher average household incomes. No statistically significant correlation was found between solar or woody biomass rebates and household income.
1. INTRODUCTION
As the need to decarbonize the United States (U.S.) energy system grows, more state energy agencies are offering technology incentive programs to promote increased renewable energy generation, environmental quality, and regional development, among other policy goals (DSIRE, 2020). The Maryland Energy Administration (MEA)'s Residential Clean Energy Rebate Program (active 2005 - present) and Clean Burning Wood and Pellet Stove RebateProgram (active 2012 - present) are two programs seeking to accomplish these objectives by offering consumers rebates for installing qualifying residential renewable energy technologies including solar panels, woody biomass heating systems, and geothermal systems.
Rebates programs assume that novel energy technologies debut at a higher price than established energy technologies, but their prices fall as popularity with consumers increases. Incentives are presumed to make novel low-carbon technologies more attractive to potential buyers, accelerating their demand and adoption rate. Consequently, energy technology rebate programs are often judged on the principle of additionality—did the program result in more renewable energy generation/carbon reduction than would have occurred in its absence? An additional metric of program success is justice—did the program serve communities/regions equitably? Both metrics are important, as the need to decarbonize rapidly for the sake of the climate must be balanced with the need to secure public acceptance.
This research focuses on these metrics by analyzing the rebate distribution patterns of MEA's two residential renewable energy rebate programs. The analysis uses MEA program data collected over a period of 15 years (February 2005-June 2020) to evaluate how the distribution of technology incentives across different energy resource types (solar, geothermal, and woody biomass) have thus far aligned with MEA's desire to distribute renewable energy program funds across the state equitably while also maximizing carbon reduction and environmental benefit.
Of special interest in this study is the magnitude of divergence in the distribution patterns of wood and pellet stove and solar and geothermal rebates, given the large gap in cost for these systems. The MEA's rebate programs use a tiered rebate structure, wherein solar PV rebates are set at $1,000; solar hot water rebates $500; geothermal systems $3,000; wood stoves $500, and pellet stoves $700, as of June 2020. The rebate amounts reflect that the total cost of residential solar PV and geothermal energy systems are typically six to eight times the cost of a wood or pellet stove installation, according to project cost data.
Because the MEA neither excludes higher income households from participation nor provides higher rebates to lower income households, we hypothesized that rebates for more expensive renewable technologies will be claimed by more economically secure households. Moreover, we anticipated that the different physical characteristics of renewable energy systems will influence their adoption rate in specific regions. For example, we expected wood stoves to be more popular in more forested rural areas where fuel is more readily available. This study investigates whether these expectations align with the actual rebate distribution patterns to date.
II. DATA AND METHODS
Renewable Technology Rebates Dataset
The MEA rebate dataset used in this study combined publicly available rebate program datasets accessed online through the state's Open Data Portal with additional program data for requested and obtained directly from MEA. The result is a robust dataset that contains a total of 27,728 technology rebates. Solar rebates comprise 66% of the dataset, geothermal rebates 20%, and wood and pellet rebates 14%. (Note: The Clean Burning Wood and Pellet program launched six years after the Residential Clean Energy program with solar and geothermal technologies). Approximately 84% of the wood burning device rebates were awarded to pellet stoves.
​Table 1: This table summarizes the awards granted to Maryland zip codes from January 2005 to June 2020. Standard deviation (s.d.) measures the degree of variation in the dataset. A low s.d. indicates more value clustering around the mean, while a high s.d. indicates higher dispersion across the range. Solar rebates had the most variation in terms of the number of rebates granted to a zip code.
Analysis Methods
Quantitative spatial analysis techniques were used to estimate the degree of correlation between the number of renewable energy incentives granted to a zip code and the presence of potential explanatory variables, such as household income. The zip code was used as the unit of analysis because it is the most precise scale for which rebate and other data is currently available. Ideally, the study would have been conducted at an even more granular scale (Census tract; neighborhood) to assess whether rebates are reaching disadvantaged communities. Household income, housing stock age, and electricity price, and other social and economic variables were selected to characterize areas of relative privilege within the state.
The analysis utilized two methods: hot spot/cold spot analysis and ordinary least squares (OLS) regression. Rebate hot spots and cold spots were estimated using the Optimized Hot Spot Analysis function in ArcGIS 10.7 software. This tool identifies spatial clusters with high and low numbers of rebates and calculates the confidence level of statistical significance using z-scores and p-values.[1] In the maps presented in this report, statistically significant hot spots (zip codes that received many rebates) are depicted in orange/red and statistically significant cold spots (zip codes that received few rebates) in shades of blue. The darker the hue, the greater the probability that the region constitutes a hot or cold spot.
To supplement the hot spot/cold spot analysis, an ordinary least squares (OLS) regression model was created to assess the statistical relationship between rebate count and explanatory variables. OLS generates a regression equation to represent the degree a selected variable, such as household income or electricity price, promote a positive or negative change in the number of incentives awarded (dependent variable). Each explanatory variable is assigned a regression coefficient (β) indicating the sign (positive or negative) and strength of its relationship to the dependent variable.
[1] The z-score indicates the intensity of the clustering (higher or lower z-scores signal higher clustering; z-scores near 0 signal low or no clustering), while p-scores measure the probability that the observed spatial pattern was created by a random process (small p-values indicates it is unlikely that the pattern can be attributed to random process).
III. RESULTS
Hot spot/cold spot analysis
The results of the hot spot/cold spot analysis are depicted in a series of maps. The first three maps show rebate hot spots and cold spots for each technology type: solar, geothermal, and woody biomass. The fourth and final map contains the rebate hot spots for all technology types.
Figure 1: Solar rebate hot spots (red) and cold spots (blue)
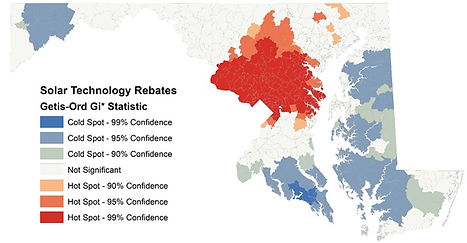
The hot spot/cold spot analysis found that solar rebates were more likely to be awarded in Montgomery, Price George's, and Howard Counties and less likely to be awarded in the Eastern Shore, Southern Maryland, and Western Panhandle (Alleghany and Garrett Counties).
Figure 2: Geothermal rebate hot spots and cold spots
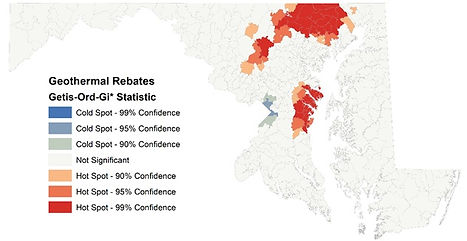
Geothermal rebates were more likely to be awarded in central, northeast, and southeast Maryland, and less likely to be awarded near Washington DC.
​Table 1: This table summarizes the awards granted to Maryland zip codes from January 2005 to June 2020. Standard deviation (s.d.) measures the degree of variation in the dataset. A low s.d. indicates more value clustering around the mean, while a high s.d. indicates higher dispersion across the range. Solar rebates had the most variation in terms of the number of rebates granted to a zip code.
Figure 3: Wood and pellet rebate hot spots and cold spots

Wood and pellet rebates were more likely to be awarded in Harford County and eastern Southern Maryland (Calvert County), but less likely to be awarded in Montgomery and Howard Counties.
Figure 4: All rebates hotspots (99% statistically significant confidence level)
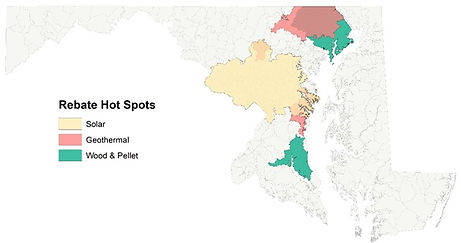
Solar and woody biomass rebate hotspots have no overlap with each other, while geothermal hotspots overlapped greatly with the wood and pellet hotspot in Harford County and, to a lesser extent, the solar rebate hotspot in the central part of the state.
OLS Regression
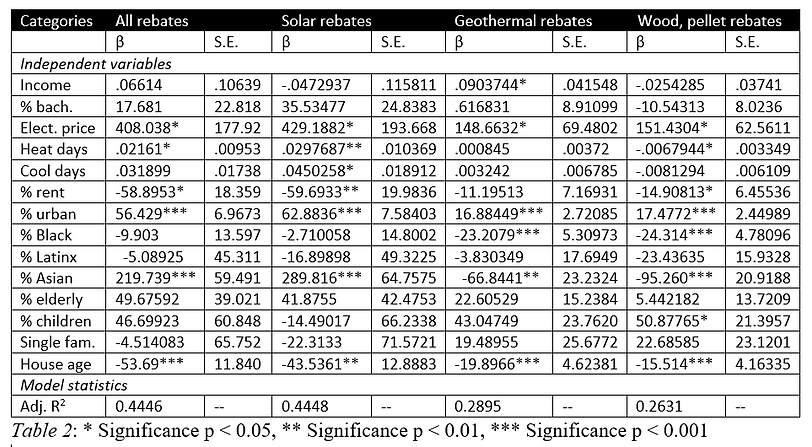
The symbol β denotes the coefficient for each independent variable, while S.E. represents the standard error term. Adjusted R2 measures how well the OLS model explains the variation in the dependent variable overall. The closer the R-squared value is to 1.0 (100%), the more the set of variables in the OLS model collectively explain rebate distribution patterns. Lower R-squared values signify that the set of variables chosen for the model do not explain the rebate distribution patterns fully and additional explanatory variables must be found.
In this case, the adjusted R-squared values ranged from 0.26 for the wood and pellet stove grant model to 0.44 for all rebates. The OLS regression explains nearly one half of the variation in the distribution of residential low-carbon technology incentives (R2 = 0.45), which suggests there are other variables not included in this study that account for some of the variation in the rebate distribution.
The OLS regression model for solar, wood and pellet, and geothermal rebates (the full rebate dataset) uncovered that the prevalence of renters and older housing stock were negatively correlated with rebate counts, while urbanity (percentage of zip code classified as urban), percent of Asian-identifying residents, higher electricity prices, and heating degree days were positively correlated. Put differently, the model shows rebates are more likely to be awarded to ‘urban'[1] and warmer zip codes with a higher than average share of Asian residents, higher than average electricity prices, fewer renters (more homeowners), and newer housing stock.
Surprisingly, the OLS models did not find statistically significant relationships between average household income and the presence of solar or woody biomass rebates. A statistically significant positive relationship was found between income and geothermal rebates, which may reflect the high total cost of residential geothermal installations.
​
Supplementary analyses
While the OLS regression offers some insight the rebate distribution patterns for different technologies, the model does not explain all the variation in the dataset. Additional maps were created in ArcGIS to better understand how rebate distribution patterns relate spatially to other key variables, namely (1) population; (2) household income; (3) primary household heating fuel; and (4) forest cover. The results of these bi-variate analyses are presented in a series of maps that compare technology rebate distribution patterns with distributions of the selected variables.
​
1. Population
The three maps in this series compare population per square mile with hot and cold spots for various rebate types. Darker areas on these maps indicate a higher population density. Population data is from the 2010 U.S. Decennial Census.
Figure 5: Wood and pellet rebate hot and cold spots and population density
[1] Note: The U.S. Census definition of urban is very broad and encompasses tracts with 50,000 or more people, not only major cities like Baltimore. Over 70% of Maryland zip codes are classified as over 50% urban.
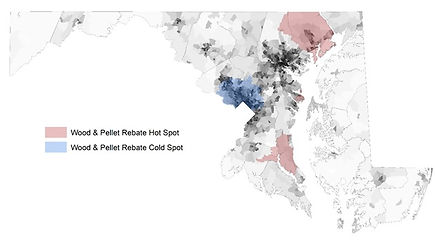
Wood and pellet rebate hot spots are not located in highly populated areas of the state. The lack of a hot spot in the most densely populated areas (DC suburbs and Baltimore) suggests program directives regarding restricting participation to utility gas-using homes are likely being followed.
Figure 6: Geothermal rebate hot spots and population density
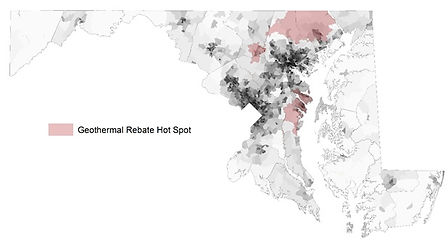
Geothermal systems usually need a large back yard or property which can be dug up, making them more unlikely to be found in urban areas and more densely settled neighborhoods.
Figure 7:Solar rebate hot and cold spots and population density
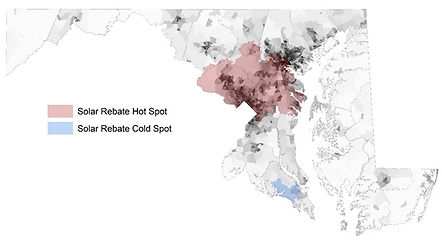
The solar rebate hot spot lies in a densely populated area of the state.
2. Household Income
This series of maps visualize the relationship between rebate hot spots and median household income. Income data comes from the 2019 American Community Survey (ACS).
Figure 8: Wood and pellet rebate hot and cold spots and median household income
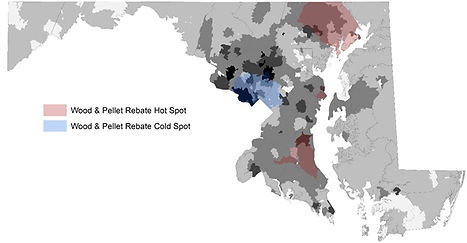
​Wood and pellet rebate clusters tend to lie within moderate income areas.
Figure 9: Geothermal rebate hot spots and median household income

The geothermal rebate hot spots are in regions with a mix of moderate and high incomes.
Figure 10: Solar rebate hot and cold spots and median household income
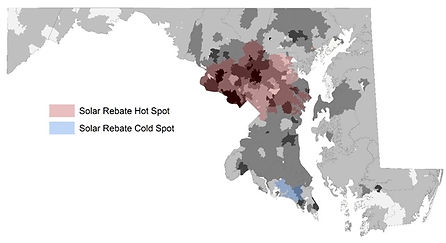
The solar rebate hotspot extends into lower-income neighborhoods surrounding DC, but also includes more affluent areas.
3. Primary Household Heating Fuel
The two maps illustrate the location of woody biomass and solar rebate hot spots relative to the number of Maryland residences that rely mainly on these heating fuels. Primary heating fuel data is taken from the 2018 ACS. Note: The Census does not currently track the number of homes heating primarily with geothermal systems, so this relationship could not be mapped.
Figure 11: Wood and pellet rebate hot spots and wood and pellet heating households
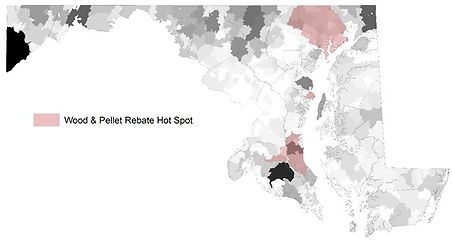.jpg)
Homes that primarily heat are concentrated in Western Maryland, a part of the state that did not receive a large portion of rebates.
Figure 12: Solar rebate hot spot and solar heating households

The solar rebate hotspot is located near a prominent solar residential heating hot spot.
4. Forest Cover
The map below shows the relationship between rebate hotspots and forested land. Data on forest cover comes from the Maryland Department of Planning.
Figure 13: Rebate hotspots and forest canopy
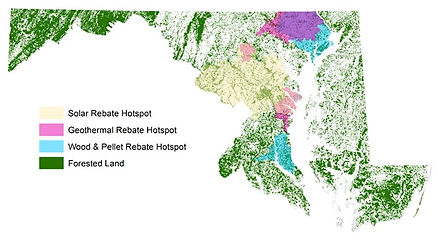
Wood and pellet and geothermal rebates tend to cluster in more forested areas that solar rebates.
Figure 10: Solar rebate hot and cold spots and median household income
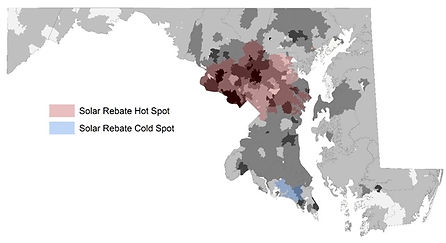
The solar rebate hotspot extends into lower-income neighborhoods surrounding DC, but also includes more affluent areas.
IV. CONCLUSION
Results of this study show that the program's rebate distributions patterns conformed to some expectations but subverted others. The hot spot/cold spot analysis confirmed the hypothesis that rebate distribution patterns varied across technology types. As suspected, more solar rebates were claimed in the more affluent and less forested parts of the state. Wood and pellet rebates were less likely to be awarded in Montgomery and Howard Counties where a greater number of residents have access to utility gas heat. Geothermal rebates were more likely to be awarded in central, northeast, and southeast portions of the state where the population density tends to be lower (though not as low as on the Eastern Shore) and median incomes higher.
The OLS regression model found that rebates for all technology types were less likely to be awarded to zip codes with a higher percentage of renters and older construction homes and more likely to awarded to Maryland zip codes with a greater percentage of Asian-identifying residents, higher electricity prices, and a higher number of warmer days. On the whole, these trends conform to expectations concerning low-carbon technology adoption as stated in the research literature.
A more suprising finding was the absence of a statistically significant correlation between solar or woody biomass rebates and median household income. Overall, fifteen years of program data indicates MEA residential low-carbon technology rebates are not disproportionately flowing to higher income regions, an indicator of a more equitable energy transition policy. On the other hand, rebates have tended to cluster in moderate to high-income areas, and the program could do a better job of reaching lower income communities in Western Maryland and the Eastern Shore. Follow-up studies could test whether these trends hold true for other scales, such as county or Census Tract, as well as other U.S. states.
Acknowledgements: I would like to thank the Edna Bailey Sussman Foundation for funding this research, Garry Aime and Jennifer Gallicchio for their help in obtaining MEA program data, and John Ackerly and Jonathan Kays for their excellent suggestions and corrections on earlier drafts. I alone am responsible for any remaining errors.
​
About the author: Melissa Bollman is a PhD candidate at Clark University's Graduate School of Geography in Worcester, Massachusetts. Her dissertation research focuses on the contested role of residential woody biomass technologies in U.S. clean energy transition policy. She served as a research fellow and program manager for the Alliance for Green Heat from 2011 to 2013.
V. APPENDIX - WOOD STOVE REBATE AND RETAIL LOCATIONS
An additional variable that could explain wood and pellet rebate distribution patterns is proximity to wood and pellet stove retailers. Because retailers play an important role in program outreach, we would expect more stove rebates to be claimed near stores that sell eligible stoves. Most wood and pellet stove retailers are located in the Central and Southern Maryland, and there does appear to be some clustering of rebates around many, but not all, retail locations.
Figure 14: Wood and pellet rebates and retailers
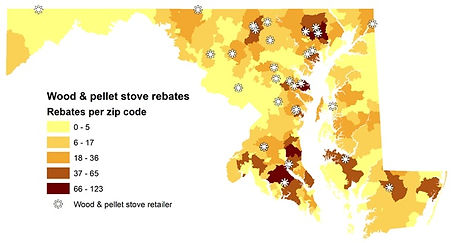.jpg)
The absence of rebates clusters near some retailers could indicate that most customers have access to natural gas (which would leave them ineligible for a rebate, according to program criteria), among other possibilities.
​
The presence of rebate clusters could indicate a higher level of free riders (homeowners who would have purchased the same technology in the absense of the program) but also assures stoves were professionally installed and permitted. Without the program's requirements, some buyers would have likely installed the device themselves without a permit. The program also may have influenced the purchase of more fuel-efficient stoves, which tend to be cleaner, as rebates were not available for stoves under 70% efficiency.
Figure 15: Wood and pellet stove retailers near rebate clusters

Two Southern Maryland retailers—Superior Fireplace and Hot Tubs and SOMD Hearth—may have been active promoters and/or beneficiaries of the wood and pellet rebate program, as well as a group of Courtland Hardware stores in Hartford County.